|
|
|
本文已被:浏览 6384次 下载 4016次 |
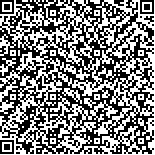 码上扫一扫! |
机器学习中成员推理攻击和防御研究综述 |
牛俊,马骁骥,陈颖,张歌,何志鹏,侯哲贤,朱笑岩,伍高飞,陈恺,张玉清 |
|
(西安电子科技大学计算机科学与技术学院 西安 中国 710071;国家计算机网络入侵防范中心 中国科学院大学 北京 中国 101408;海南大学网络空间安全学院 海口 中国 570228;国家计算机网络入侵防范中心 中国科学院大学 北京 中国 101408;国家计算机网络入侵防范中心 中国科学院大学 北京 中国 101408;西安电子科技大学广州研究院 广州 中国 510555;国家计算机网络入侵防范中心 中国科学院大学 北京 中国 101408;西安邮电大学网络空间安全学院 西安 中国 710121;国家计算机网络入侵防范中心 中国科学院大学 北京 中国 101408;西安电子科技大学网络与信息安全学院 西安 中国 710126;西安电子科技大学通信工程学院 西安 中国 710071;中国科学院信息工程研究所 信息安全国家重点实验室 北京 中国 100195;中国科学院大学 网络空间安全学院 北京 中国 100195;国家计算机网络入侵防范中心 中国科学院大学 北京 中国 101408;海南大学网络空间安全学院 海口 中国 570228;西安邮电大学网络空间安全学院 西安 中国 710121;西安电子科技大学网络与信息安全学院 西安 中国 710126) |
|
摘要: |
机器学习被广泛应用于各个领域, 已成为推动各行业革命的强大动力, 极大促进了人工智能的繁荣与发展。同时, 机器学习模型的训练和预测均需要大量数据, 而这些数据可能包含隐私信息, 导致其隐私安全面临严峻挑战。成员推理攻击主要通过推测一个数据样本是否被用于训练目标模型来破坏数据隐私, 其不仅可以破坏多种机器学习模型(如, 分类模型和生成模型)的数据隐私, 而且其隐私泄露也渗透到图像分类、语音识别、自然语言处理、计算机视觉等领域, 这对机器学习的长远发展产生了极大的安全威胁。因此, 为了提高机器学习模型对成员推理攻击的安全性, 本文从机器学习隐私安全攻防角度, 全面系统性分析和总结了成员推理攻击和防御的基本原理和特点。首先, 介绍了成员推理攻击的定义、威胁模型, 并从攻击原理、攻击场景、背景知识、攻击的目标模型、攻击领域、攻击数据集大小六个方面对成员推理攻击进行分类, 比较不同攻击的优缺点; 然后, 从目标模型的训练数据、模型类型以及模型的过拟合程度三个角度分析成员推理攻击存在原因, 并从差分隐私、正则化、数据增强、模型堆叠、早停、信任分数掩蔽和知识蒸馏七个层面对比分析不同防御措施; 接着, 归纳总结了成员推理攻击和防御常用的评估指标和数据集, 以及其在其他方面的应用。最后, 通过对比分析已有成员推理攻击和防御的优缺点, 对其面临的挑战和未来研究方向进行了展望。 |
关键词: 机器学习 成员推理攻击 隐私安全 防御措施 |
DOI:10.19363/J.cnki.cn10-1380/tn.2022.11.01 |
投稿时间:2022-07-04修订日期:2022-10-06 |
基金项目:本课题得到国家自然科学基金项目(No. U1836210, No. 61772406); 海南省重点研发计划项目(No. ZDYF202012); 陕西省自然科学基础研究计划资助项目(No. 2021JQ-192 ); 中央高校基本科研业务费专项资金(No. JB211508)资助。 |
|
A survey on membership inference attacks and defenses in Machine Learning |
NIU Jun,MA Xiaoji,CHEN Ying,ZHANG Ge,HE Zhipeng,HOU Zhexian,ZHU Xiaoyan,WU Gaofei,CHEN Kai,ZHANG Yuqing |
School of Computer Science and Technology, Xidian University, Xi'an 710071, China;National Computer Network Instrusion Protection Center, University of Chinese Academy of Sciences, Beijing 101408, China;College of Cyberspace Security, Hainan University, Haikou 570228, China;National Computer Network Instrusion Protection Center, University of Chinese Academy of Sciences, Beijing 101408, China;National Computer Network Instrusion Protection Center, University of Chinese Academy of Sciences, Beijing 101408, China;School of Guangzhou Research Institute, Xidian University, Guangzhou 510555, China;National Computer Network Instrusion Protection Center, University of Chinese Academy of Sciences, Beijing 101408, China;School of Cyberspace Security, Xi'an University of Posts & Telecommunications, Xi'an 710121, China;National Computer Network Instrusion Protection Center, University of Chinese Academy of Sciences, Beijing 101408, China;School of Cyber Engineering, Xidian University, Xi'an 710126, China;School of Telecommunications Engineering, Xidian University, Xi'an 710071, China;SKLOIS, Institute of Information Engineering, Chinese Academy of Sciences, Beijing 100195, China;School of Cyber Security, University of Chinese Academy of Sciences, Beijing 100195, China;National Computer Network Instrusion Protection Center, University of Chinese Academy of Sciences, Beijing 101408, China;College of Cyberspace Security, Hainan University, Haikou 570228, China;School of Cyberspace Security, Xi'an University of Posts & Telecommunications, Xi'an 710121, China;School of Cyber Engineering, Xidian University, Xi'an 710126, China |
Abstract: |
The newly emerged machine learning (ML) methods have been widely applied to various applications, and have become a strong driving force to revolutionize a wide range of industries, which have greatly promoted the prosperity and development of artificial intelligence. Meanwhile, the training and inference of the machine learning model are based on a large amount of data, which always contains some private information. And the privacy and security of the ML has faced serious challenges. Membership inference attacks (MIAs) mainly aim to infer whether a data record was used to train a target model or not. MIAs have not only been shown to be effective on various ML models (e.g., classification models and generative models), but also have been penetrated into the fields of image classification, speech recognition, natural language processing, computer vision and so on, which creates a great security threat to the long-term development of machine learning. Therefore, in order to better improve the security of ML models for membership inference attacks, in this paper, we systematically introduce and analyze the basic principles and characteristics of the MIAs and their defenses from a ML attack-defense perspective. Firstly, we introduce the definitions and threat models of the MIAs, and classify these MIAs from six different perspectives such as attacks’ principles, scenarios, background knowledge, target models, fields and the size of attack datasets, and we compare their advantages and disadvantages. Secondly, we summary the reasons caused the MIAs from three aspects, namely diversity of training data, types of target models and overfitting of target models. Thirdly, we survey defensive techniques for MIAs as well as their characteristics by differential privacy, regularization, data argumentation, model stacking, early stopping, confidence score masking and knowledge distillation. Futhermore, we institute the evaluation metrics and datasets used in MIAs, and the other applications of the MIAs. Finally, by comparing and analyzing the existing MIAs and their defenses, we discuss the challenges and future research directions. |
Key words: machine learning membership inference attacks privacy & security defensive techniques |
|
|
|
|
|
|