引用本文: |
-
钱亚冠,刘新伟,顾钊铨,王滨,潘俊,张锡敏.一种基于二维码对抗样本的物理补丁攻击[J].信息安全学报,2020,5(6):75-86 [点击复制]
- QIAN Yaguan,LIU Xinwei,GU Zhaoquan,WANG Bin,PAN Jun,ZHANG Ximin.QR Code Based Patch Attacks in Physical World[J].Journal of Cyber Security,2020,5(6):75-86 [点击复制]
|
|
本文已被:浏览 9193次 下载 7233次 |
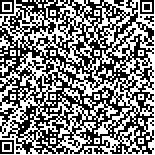 码上扫一扫! |
一种基于二维码对抗样本的物理补丁攻击 |
钱亚冠1, 刘新伟1, 顾钊铨2, 王滨3, 潘俊1, 张锡敏1
|
|
(1.浙江科技学院大数据学院, 杭州 中国 310023;2.广州大学网络空间先进技术研究院, 广州 中国 510006;3.杭州海康威视网络与信息安全实验室, 杭州 中国 310051) |
|
摘要: |
深度学习技术在图像识别领域已经得到广泛应用,识别准确率超过人类平均水平。然而最近的研究表明,深度神经网络的性能会因对抗样本的存在而大幅降低。攻击者通过在待识别的图像中添加精心设计的微小扰动,误导分类器做出错误预测。另一个方面,在数字空间生成的扰动也能够转移到物理空间并用于攻击。为此,本文提出了一种基于二维码对抗样本的物理补丁攻击方法。将生成的二维码贴在道路交通标志表面的指定位置,使得分类器输出错误的分类。实验结果表明了本文方法的有效性,同时,将数字空间生成的对抗样本用于物理空间中的交通标志攻击,仍可以保持较高的成功率。 |
关键词: 深度学习 对抗样本 二维码 补丁攻击 |
DOI:10.19363/J.cnki.cn10-1380/tn.2020.11.07 |
投稿时间:2019-12-29修订日期:2020-01-05 |
基金项目:本课题得到科技部重点研发项目(No.2018YFB2100400);国家自然科学基金资助项目(No.61902082);浙江省自然科学基金资助项目(No.LY17F020011);浙江省公益技术应用研究项目(No.LGF20F020007,No.LGG19F030001)资助。 |
|
QR Code Based Patch Attacks in Physical World |
QIAN Yaguan1, LIU Xinwei1, GU Zhaoquan2, WANG Bin3, PAN Jun1, ZHANG Ximin1
|
(1.School of Sugon Big Date Science, Zhejiang University of Science and Technology, Hangzhou 310023, China;2.Cyberspace Institute of Advanced Technology (CIAT), Guangzhou University, Guangzhou 510006, China;3.Network and Information Security Laboratory of Hangzhou Hikvision Digital Technology Co, Ltd. Hangzhou 310051, China) |
Abstract: |
Deep learning technology has been widely used in the field of image recognition, and the recognition accuracy is higher than the average level of human beings. However, recent studies have shown that the performance of deep neural network will be greatly reduced due to the presence of adversarial examples. The attacker misleads the classifier to make false prediction by adding a small disturbance to the image to be recognized. On the other hand, the disturbance generated in the digital space can also be transferred to the physical space and used for attack. For this reason, this paper proposes a physical patch attack method based on two-dimensional code antagonism samples, which pastes the generated QR code on the designated position of the road traffic sign surface, making the classifier output the wrong classification. The experimental results show the effectiveness of this method. At the same time, using the counter examples generated in digital space to attack traffic signs in physical space can still maintain a high success rate. |
Key words: deep learning adversarial examples QR code patch attack |