引用本文: |
-
王尚,李昕,宋永立,苏铓,付安民.基于自定义后门的触发器样本检测方案[J].信息安全学报,2022,7(6):48-61 [点击复制]
- WANG Shang,LI Xin,SONG Yongli,SU Mang,FU Anmin.A Trigger Sample Detection Scheme Based on Custom Backdoor Behaviors[J].Journal of Cyber Security,2022,7(6):48-61 [点击复制]
|
|
|
|
本文已被:浏览 6933次 下载 4792次 |
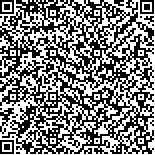 码上扫一扫! |
基于自定义后门的触发器样本检测方案 |
王尚1,2, 李昕3, 宋永立3, 苏铓1, 付安民1,2
|
|
(1.南京理工大学计算机科学与工程学院 南京 中国 210094;2.中国科学院信息工程研究所信息安全国家重点实验室 北京 中国 100093;3.北京计算机技术及应用研究所 北京 中国 100036) |
|
摘要: |
深度学习利用强大的特征表示和学习能力为金融、医疗等多个领域注入新的活力, 但其训练过程存在安全威胁漏洞, 攻击者容易通过操纵训练集或修改模型权重执行主流后门攻击: 数据中毒攻击与模型中毒攻击。两类攻击所产生的后门行为十分隐蔽, 后门模型可以保持干净样本的分类精度, 同时对嵌入攻击者预定义触发器的样本呈现定向误分类。针对干净样本与触发器样本在拟合程度上的区别, 提出一种基于自定义后门行为的触发器样本检测方案 BackDetc, 防御者自定义一种微小触发器并执行数据中毒攻击向模型注入自定义的后门, 接着通过嵌入自定义触发器设计一种输入样本扰动机制, 根据自定义触发器的透明度衡量输入样本的拟合程度, 最终以干净样本的拟合程度为参照设置异常检测的阈值, 进而识别触发器样本, 不仅维持资源受限用户可负担的计算开销, 而且降低了后门防御假设, 能够部署于实际应用中, 成功抵御主流后门攻击以及威胁更大的类可知后门攻击。在 MNIST、 CIFAR-10 等分类任务中, BackDetc 对数据中毒攻击与模型中毒攻击的检测成功率均高于目前的触发器样本检测方案, 平均达到 99.8%以上。此外, 论文探究了检测假阳率对检测性能的影响, 并给出了动态调整 BackDetc 检测效果的方法, 能够以 100%的检测成功率抵御所有分类任务中的主流后门攻击。最后, 在 CIFAR-10 任务中实现类可知后门攻击并对比各类触发器样本检测方案, 仅有 BackDetc 成功抵御此类攻击并通过调整假阳率将检测成功率提升至 96.2%。 |
关键词: 深度学习 后门攻击 自定义后门 拟合程度 触发器样本 |
DOI:10.19363/J.cnki.cn10-1380/tn.2022.11.03 |
投稿时间:2022-06-20修订日期:2022-08-04 |
基金项目:本课题得到国家自然科学基金(No. 62072239), 江苏省自然科学基金(No. BK20211192), 信息安全国家重点实验室开放基金(No.2021-MS-07)资助。 |
|
A Trigger Sample Detection Scheme Based on Custom Backdoor Behaviors |
WANG Shang1,2, LI Xin3, SONG Yongli3, SU Mang1, FU Anmin1,2
|
(1.School of Computer Science and Engineering, Nanjing University of Science and Technology, Nanjing 210094, China;2.State Key Laboratory of Information Security, Institute of Information Engineering, Chinese Academy of Sciences, Beijing 100093, China;3.Beijing Institute of Computer Technology and Application, Beijing 100036, China) |
Abstract: |
Deep learning leverages powerful feature representation and learning capabilities to breathe new life into various fields such as finance and healthcare, but the training process is vulnerable to security threats, easily introducing mainstream backdoor attacks through manipulating the training data set or modifying model weights, including data poisoning attack and model poison attack. The backdoor implanted by both types of backdoor attacks is great stealthy, the backdoored model can maintain the clean data accuracy, while presenting targeted misclassification for samples embedded with the attacker-specific triggers. This paper proposes a custom backdoor behavior-based trigger samples detection scheme BackDetc, focusing on the essential difference on the fit degree between clean samples and trigger samples. It injects custom backdoors into the model through tiny defender-custom triggers, proposing an input sample perturbation mechanism by embedding these custom triggers. We measure the fit degree of inputs adopting the transparency of the custom trigger, and calculate the threshold of anomaly detection with the fit degree of clean samples as a reference, identifying these samples with attacker-specific triggers. In this way, BackDetc not only holds the affordable overhead for resource limited users, but reduces the strength of backdoor defense assumption, being deployed in various real-world applications and being effective for mainstream backdoor attacks as well as more threatening source-specific backdoor attacks. In experiments, the BackDetc is deployed on MNIST, CIFAR-10 classification tasks, outperforming other existing trigger samples detection schemes on detection success rate when facing data poisoning attack and model poison attack, with an average of over 99.8%. Then, the influence of the detection false positive rate is explored on the detection performance, giving the capability of dynamically adjusting the detection effect of BackDetc, displaying 100% detection success rate on all tasks when encountering two mainstream backdoor attacks. Meanwhile, in the CIFAR-10 task, a source-specific backdoor attack is implemented to evaluate various trigger samples detection schemes, only BackDetc successfully resists such the attack and increases the detection success rate to 96.2% by adjusting the false positive rate. |
Key words: deep learning backdoor attack customize backdoor fit degree trigger samples |
|
|
|
|
|