引用本文: |
-
黄珊珊,金鑫,吴楠,江倩.结合频域信息与对抗网络的虚假图像检测[J].信息安全学报,2023,8(6):37-47 [点击复制]
- HUANG Shanshan,JIN Xin,WU Nan,JIANG Qian.Fake Image Detection Combining Frequency Domain Information and Adversarial Network[J].Journal of Cyber Security,2023,8(6):37-47 [点击复制]
|
|
|
|
本文已被:浏览 6417次 下载 5031次 |
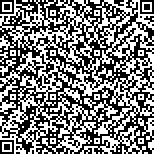 码上扫一扫! |
结合频域信息与对抗网络的虚假图像检测 |
黄珊珊1,2, 金鑫2,3, 吴楠2,3, 江倩2,3
|
|
(1.重庆大学大数据与软件学院 重庆 中国 401331;2.跨境网络空间安全教育部工程研究中心 昆明 中国 650504;3.云南大学软件学院 昆明 中国 650504) |
|
摘要: |
随着深度学习方法的发展, 深度造假(Deepfake)技术越发成熟。大量近似真实自然的图像涌入人们的生活, 在满足个人娱乐兴趣的同时, Deepfake技术的滥用对个人隐私、经济市场乃至国家安全构成了潜在威胁。因此,针对虚假图像的检测方法亟待研究。现有的虚假图像检测技术大多存在准确率低、泛化性差、鲁棒性不足的问题, 因此, 本文从Deepfake技术的图像生成机制出发, 对生成的虚假图像存在缺陷进行分析, 并提出了一种基于生成对抗网络的虚假图像检测模型。该模型利用离散傅里叶变换方法将图像从图像域转换到频域, 并将U-Net结构和谱归一化引入鉴别器; 利用生成对抗网络优异的特征学习和提取能力, 实现了虚假图像的模式分类。此外, 一种新颖的复合损失函数被提出, 以增强模型检测性能。提出的方法分别在7个单独数据集和1个混合数据集上进行实验验证, 并采用3种实验指标进行模型性能分析。本文方法在单独数据集上最高可达到100%准确率, 最低准确率也可达88.53%; 模型检测召回率, 精确率和F1分数平均分别可达98.17%, 98.25%, 98.19%。此外, 无论是在混合数据集, 还是在模型未知的跨数据集上, 提出方法都能获得良好的模型检测性能。即使在图像压缩的情况下, 本文方法仍然具有较强的鲁棒性。实验与理论结果表明, 与现有先进的虚假图像检测方法相比, 本文方法是一种有效且具有良好泛化性和鲁棒性的虚假图像检测方法。 |
关键词: 信息安全 图像处理 深度造假 虚假图像检测 生成对抗网络 |
DOI:10.19363/J.cnki.cn10-1380/tn.2023.11.04 |
投稿时间:2022-03-17修订日期:2022-05-27 |
基金项目:本课题得到国家自然科学基金(No. 62002313, No. 62101481, No. 62261060); 云南省重点研发领域计划项目(No. 202001BB050076); 云南省基础研究计划项目(No. 202201AU070033, No. 202201AT070112, No. 202301AU070210, No. 202301AW070007); 云南省科技厅重大科技专项(No. 202202AD080002); 云南省软件工程重点实验室开放基金资助项目(No. 2020SE408); 云南大学第 11 届研究生科研创新项目(No. 2019164)资助。 |
|
Fake Image Detection Combining Frequency Domain Information and Adversarial Network |
HUANG Shanshan1,2, JIN Xin2,3, WU Nan2,3, JIANG Qian2,3
|
(1.School of Big Data & Software Engineering, Chongqing University, Chongqing 401331, China;2.Engineering Research Center of Cyberspace, Kunming 650504, China;3.School of Software, Yunnan University, Kunming 650504, China) |
Abstract: |
With the development of deep learning methods, deepfake technique has become more and more mature. With the flood of plausible images poured into people's insight, as well as satisfying personal entertainment interests, the misuse of deepfake technique poses a potential threat to personal privacy, economic markets, and even national security. Therefore, it is urgent to study fake image detection methods. Most of the existing methods have the problem of low accuracy, poor generalization, and lack of robustness. Therefore, this paper analyzes the defects of generated false images from the image generation mechanism of deepfake technique, and proposes a fake image detection model based on generative adversarial networks. This model uses the discrete Fourier transform method to transform the image from the image domain to the frequency domain, and introduces the U-Net structure and spectral normalization into the discriminator; the excellent feature learning and extraction capability of generative adversarial networks are utilized to achieve pattern classification of fake images. In addition, a novel composite loss function is proposed to enhance the model detection performance. The proposed method is validated on seven individual datasets and one hybrid dataset, respectively, and three experimental metrics are used for model performance analysis. Compared with the existing advanced methods, the proposed method can achieve the highest accuracy of 100% and the lowest accuracy of 88.53% on separate datasets. The average recall, precision, and F1 score are 98.17%, 98.25%, and 98.19%, respectively. In addition, the proposed method achieves good model detection performance regardless of the hybrid dataset or the cross-dataset where the model is unknown. Further, even in the case of image compression, the method in this paper still has strong robustness. In summary, compared with existing state-of-the-art fake image detection methods, the proposed method is an effective fake image detection method with good generalization and robustness. |
Key words: cyber security deepfake fake image detection generative adversarial networks |
|
|
|
|
|