引用本文: |
-
程鑫,王金伟,王昊,罗向阳,李晓龙,朱国普,马宾.JPEG量化步长估计综述[J].信息安全学报,2024,9(4):150-162 [点击复制]
- CHENG Xin,WANG Jinwei,WANG Hao,LUO Xiangyang,LI Xiaolong,ZHU Guopu,MA Bin.A Survey on Estimation of JPEG Quantization Step[J].Journal of Cyber Security,2024,9(4):150-162 [点击复制]
|
|
|
|
本文已被:浏览 1155次 下载 859次 |
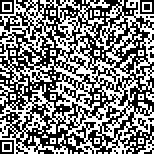 码上扫一扫! |
JPEG量化步长估计综述 |
程鑫1,2, 王金伟1,2,3, 王昊4, 罗向阳5, 李晓龙6, 朱国普7, 马宾8
|
|
(1.南京信息工程大学 数字取证教育部工程研究中心 南京 中国 210044;2.南京信息工程大学 计算机与软件学院 南京 中国 210044;3.数学工程与先进计算国家重点实验室 郑州 中国 450001;4.南京理工大学 自动化学院 南京 中国 210094;5.中国人民解放军战略支援部队信息工程大学 郑州 中国 450001;6.北京交通大学 信息科学研究所 北京 中国 100044;7.哈尔滨工业大学 网络空间安全学院 哈尔滨 中国 150001;8.齐鲁工业大学 网络空间安全学院 济南 中国 250353) |
|
摘要: |
随着 JPEG(Joint Photographic Experts Group)格式的图片在网络存储和传输中的广泛应用, 基于 JPEG 格式的篡改、 拼接等恶意操作也层出不穷, 利用 JPEG 图像特性的研究受到越来越多的关注。其中, JPEG 图像特性与量化步长息息相关。如果, JPEG 压缩后的图像以位图的形式进行保存, 研究者无法直接获取到量化步长, 从而无法有效地分析 JPEG 图像特性。因此, 对于量化步长的估计在数字图像取证领域越来越受到关注。 本文首先介绍了量化步长估计的研究背景, 并将量化步长估计问题分成两类问题: 单压缩图像的量化步长估计和重压缩图像的首次量化步长估计。其次, 在经典的 JPEG 压缩模型基础上分别了给出了这两类问题的量化步长估计模型, 并对每类问题的各种现有方法进行了详细介绍和梳理。随后, 在相同实验环境下对经典算法进行了实验, 并对经典算法进行了相应的分析与评价。 实验结果表明: 在单压缩图像的量化步长估计任务中, 现有技术相对成熟并且部分算法估计准确率高于 90%; 但是, 在对齐重压缩图像的首次量化步长估计任务中, 现有方法仅能估计低频、中频的量化步长, 并且当首次与第二次的压缩质量因子相近时, 估计准确率较低; 在非对齐重压缩图像的首次量化步长估计任务中, 由于网格偏移会增加估计量化步长的困难, 导致算法在非对齐重压缩上性能较差。最后, 指出了量化步长估计中有待进一步解决的问题和发展趋势。 |
关键词: 数字取证 JPEG压缩 量化步长估计 DCT系数分析 深度学习取证 |
DOI:10.19363/J.cnki.cn10-1380/tn.2024.07.10 |
投稿时间:2022-07-20修订日期:2022-09-25 |
基金项目:本课题得到国家自然科学基金(No. 62072250,No. 62172435,No. U20B2065);省部共建藏语智能信息处理及应用国家重点实验室(藏文信息处理教育部重点实验室)开放课题(No. 2024-Z-003);河南省网络空间态势感知重点实验室开放课题(No. HNTS2022002);中原科技创新领军人才项目(No. 214200510019);国家重点研发计划(No. 2021QY0700);江苏自然科学基金(No. BK20200750)。 |
|
A Survey on Estimation of JPEG Quantization Step |
CHENG Xin1,2, WANG Jinwei1,2,3, WANG Hao4, LUO Xiangyang5, LI Xiaolong6, ZHU Guopu7, MA Bin8
|
(1.Engineering Research Center of Digital Forensics, Ministry of Education, Nanjing University of Information Science and Technology, Nanjing 210044, China;2.Department of Computer and Software, Nanjing University of Information Science and Technology, Nanjing 210044, China;3.State Key Laboratory of Mathematical Engineering and Advanced Computing, Zhengzhou 450001, China;4.Department of Automation, Nanjing University of Science and Technology, Nanjing 210094, China;5.PLA Strategic Support Force Information Engineering University, Zhengzhou 450001, China;6.Institute of Information Science, Beijing Jiaotong University, Beijing 100044, China;7.School of Cyberspace Security, Harbin Institute of Technology, Harbin 150001, China;8.School of Cyberspace Security, Qilu University of Technology, Jinan 250353, China) |
Abstract: |
As JPEG (Joint Photographic Experts Group) format images are widely used in network storage and transmission, malicious operations such as tampering and stitching based on the JPEG format have emerged, and the research on exploiting the characteristics of JPEG images has received more and more attention. Among them, JPEG image characteristics are closely related to quantization steps. If the JPEG compressed image is saved in the bitmap form, the researcher cannot get the quantization step directly and thus cannot analyze the JPEG image characteristics effectively. Therefore, the quantization step estimation is attracting more attention in the field of digital image forensics. This paper first introduces the background of quantization step estimation and divides the problem into two issues: quantization step estimation for single compressed images and first quantization step estimation for recompressed images. Secondly, based on the classical JPEG compression model, the quantization step estimation models for these two issues are given. Various methods for each type of problem are introduced and sorted out in detail. Then, the classical algorithm experiments in the same experimental environment, and the classical algorithm is analyzed and evaluated. The experimental results show that in the quantization step estimation task of single compressed images. The existing techniques are mature, and the estimation accuracy of some algorithms is higher than 90%; however, in the first quantization step estimation task of aligned recompressed images, the current methods can only estimate the quantization steps of low and medium frequencies, and the estimation accuracy is lower when the first and second compression quality factors are similar; in the first quantization step estimation task of nonaligned recompressed images, the grid offset increases the difficulty of estimating the quantization step, resulting in poorer performance of the algorithm on nonaligned recompression. Finally, the problems to be further solved and the future development trends in the quantization step estimation task are pointed out. |
Key words: digital forensics JPEG compression quantization step estimation discrete cosine transform coefficient analysis deep learning forensics |
|
|
|
|
|