引用本文: |
-
梁思源,何英哲,刘艾杉,李京知,代朋纹,操晓春.面向大语言模型的越狱攻击与防御综述[J].信息安全学报,2024,9(5):56-86 [点击复制]
- LIANG Siyuan,HE Yingzhe,LIU Aishan,LI Jingzhi,DAI Pengwen,CAO Xiaochun.A Review of Jailbreak Attacks and Defenses for Large Language Models[J].Journal of Cyber Security,2024,9(5):56-86 [点击复制]
|
|
|
|
本文已被:浏览 2127次 下载 1012次 |
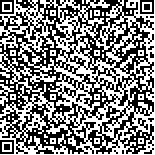 码上扫一扫! |
面向大语言模型的越狱攻击与防御综述 |
梁思源1,2, 何英哲3, 刘艾杉4, 李京知1, 代朋纹5, 操晓春5
|
|
(1.中国科学信息工程研究所 信息安全重点实验室 北京 中国 100093;2.新加坡国立大学 新加坡 新加坡 117422;3.华为北京研究所 北京 中国 100095;4.北京航空航天大学 北京 中国 100191;5.中山大学 深圳 中国 518100) |
|
摘要: |
大语言模型(Large Language Models,LLMs)由于其出色的性能表现而在各个领域被广泛使用,但是它们在面对精心构建的越狱提示时,往往会输出不正确的内容,由此引发了人们对其伦理问题和道德安全的担忧。攻击者可以在没有了解模型内部结构及安全机制的情况下,通过设计特定的提示语句引发模型生成不恰当的内容。相关领域的专业研究者在分析LLMs的潜在脆弱性后,甚至可以产生人类难以发现,并且越狱成功率极高的自动化越狱攻击方法。为了阻止LLMs的恶意越狱攻击,研究者们提出覆盖LLMs训练到部署全生命周期的防御方法以加强模型的安全性。然而,目前对于大语言模型的综述工作主要集中在越狱攻击方法,并且没有对这些技术手段的特性及关系进行详细分析。此外,对评测基准总结的忽视也限制了该领域的蓬勃发展。因此,本文拟对现有的越狱攻击与防御方法进行全面的回顾。具体而言,我们首先介绍了大语言模型与越狱攻击的相关概念及原理,解释了越狱攻击在模型安全领域的重要性和它对大型语言模型的潜在威胁。接着,从攻击的生成策略回顾了现有的越狱攻击方法,并分析了他们的优缺点,如这些攻击策略如何利用模型的漏洞来实现攻击目标。然后,本文总结了围绕LLMs各个阶段的防御策略,并提供了一个全面的评测基准,详细介绍了如何评估这些防御策略的有效性。最后结合当前面临的挑战,我们对LLMs越狱攻防的未来研究方向进行了总结和展望,指出了未来研究中需要关注的关键问题和潜在的研究方向,以促进大模型的安全与可靠性发展。 |
关键词: 越狱攻击 越狱防御 大语言模型 深度学习 可信人工智能 |
DOI:10.19363/J.cnki.cn10-1380/tn.2024.09.01 |
投稿时间:2024-04-01修订日期:2024-08-23 |
基金项目:本课题得到国家自然科学基金项目(No.62306308,No.62025604)资助。 |
|
A Review of Jailbreak Attacks and Defenses for Large Language Models |
LIANG Siyuan1,2, HE Yingzhe3, LIU Aishan4, LI Jingzhi1, DAI Pengwen5, CAO Xiaochun5
|
(1.State Key Institute of Information Engineering, Chinese Academy of Sciences, Beijing 100093, China;2.National University of Singapore, Singapore 117422, Singapore;3.Huawei Beijing Research Institute, Beijing 100095, China;4.Beihang University, Beijing 100191, China;5.Sun Yat-sen University, Shenzhen 518100, China) |
Abstract: |
Large Language Models (LLMs) have gained widespread use across various fields due to their exceptional performance. However, these models are vulnerable to generating inappropriate or incorrect outputs when exposed to carefully designed jailbreak prompts, which has sparked significant concerns about their ethical implications and safety. Attackers can exploit these models by crafting specific prompt statements that elicit unintended or harmful content, without needing to understand the models' internal workings or security mechanisms. In fact, researchers in the field have identified the inherent vulnerabilities of LLMs and developed automated jailbreak methods that are both highly effective and difficult for humans to detect. To mitigate the risks associated with these malicious jailbreak attacks, researchers have proposed comprehensive defense strategies that encompass the entire lifecycle of LLMs, from their training phases to deployment, aiming to strengthen model security. However, existing reviews of LLM security are primarily focused on describing jailbreak attack methods, often neglecting a detailed examination of the characteristics and interrelationships of these various techniques. Moreover, the lack of a thorough evaluation framework has hindered the advancement of robust defenses in this area. This paper seeks to fill that gap by providing an exhaustive review of current jailbreak attacks and defense mechanisms for LLMs. It begins with an introduction to the fundamental concepts and principles related to LLMs and jailbreak attacks, highlighting their importance in the context of model security and the potential threats they pose. The paper then delves into existing attack generation strategies, evaluating their strengths and weaknesses, particularly in how they exploit specific vulnerabilities within the models. Additionally, it offers a comprehensive summary of defense strategies across the different stages of LLMs and presents a detailed evaluation benchmark, discussing the metrics and methodologies used to assess the effectiveness of these defenses. Finally, the paper addresses the current challenges and outlines potential future research directions, emphasizing the need for ongoing attention to key issues to enhance the security and reliability of large language models. By providing a detailed and structured overview, this paper aims to guide the development of more secure, trustworthy, and ethically aligned LLMs. |
Key words: jailbreak attack jailbreak defense large language model deep learning trustworthy artificial intelligence |
|
|
|
|
|