本文已被:浏览 15050次 下载 17743次 |
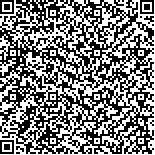 码上扫一扫! |
视听觉深度伪造检测技术研究综述 |
梁瑞刚,吕培卓,赵月,陈鹏,邢豪,张颖君,韩冀中,赫然,赵险峰,李明,陈恺 |
|
(中国科学信息工程研究所信息安全国家重点实验室 北京 中国 100093;中国科学院大学网络空间安全学院 北京 中国 100049;太原理工大学大数据学院 太原 中国 030024;中国科学院软件研究所 北京 中国 100190;中国科学院自动化研究所 北京 中国 100190) |
|
摘要: |
深度学习被广泛应用于自然语言处理、计算机视觉和无人驾驶等领域,引领了新一轮的人工智能浪潮。然而,深度学习也被用于构建对国家安全、社会稳定和个人隐私等造成潜在威胁的技术,如近期在世界范围内引起广泛关注的深度伪造技术能够生成逼真的虚假图像及音视频内容。本文介绍了深度伪造的背景及深度伪造内容生成原理,概述和分析了针对不同类型伪造内容(图像、视频、音频等)的检测方法和数据集,最后展望了深度伪造检测和防御未来的研究方向和面临的挑战。 |
关键词: 深度伪造 深度学习 生成对抗网络 |
DOI:10.19363/J.cnki.cn10-1380/tn.2020.02.01 |
Received:December 31, 2019Revised:March 04, 2020 |
基金项目:本课题得到国家重点研发项目(No.2016QY04W0805),国家自然科学基金项目(No.61728209),中国科学院青年创新促进会,北京市科技新星计划,北京市自然科学基金项目(No.JQ18011),国家前沿科技创新项目(No.YJKYYQ20170070)资助。 |
|
A Survey of Audiovisual Deepfake Detection Techniques |
LIANG Ruigang,LV Peizhuo,ZHAO Yue,CHEN Peng,XING Hao,ZHANG Yingjun,HAN Jizhong,He Ran,ZHAO Xianfeng,LI Ming,CHEN Kai |
State Key Laboratory of Information Security, Institute of Information Engineering, Chinese Academy of Sciences, Beijing 100093, China;School of Cyber Security, University of Chinese Academy of Science, Beijing 100049, China;College of Data Science, Taiyuan University of Technology, Taiyuan 030024, China;Institute of Software, Chinese Academy of Sciences, Beijing 100190, China;Institute of Automation, Chinese Academy of Sciences, Beijing 100190, China |
Abstract: |
Deep learning has been widely used in fields such as natural language processing, computer vision, and driverless vehicles, leading a new wave of artificial intelligence. Deep learning advances however have also been used to create technologies that pose potential threats to national security, social stability, and personal privacy. For example, deepfakes that have recently attracted widespread attention worldwide, which could generate seemingly realistic fake images, audio and video content. This article introduces the background of deepfakes and the principles of deepfakes creation, and then outlines and analyzes the detection methods and datasets for different types of deepfakes, including images, videos, audios, etc. Finally, the article discusses potential research directions and challenges of deepfakes detection and prevention. |
Key words: deepfakes deep learning generative adversarial network |