|
|
|
本文已被:浏览 7574次 下载 4973次 |
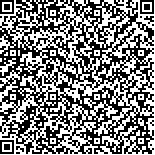 码上扫一扫! |
BATscope:比特币恶意地址及混币交易识别 |
王大宇,殷婷婷,李赟,秦嗣量,任歆,罗夏朴,王浩宇,尹霞,张超 |
|
(清华大学 计算机科学与技术系 北京 中国 100084;清华大学 网络科学与网络安全研究院 北京 中国 100084;中国科学院大学 网络空间安全学院 北京 中国 100049;厦门大学 软件工程系 厦门 中国 361005;香港理工大学 计算系 香港 中国;华中科技大学 网络空间安全学院 武汉 中国 430074) |
|
摘要: |
*比特币作为第一个也是最主流的基于区块链技术的数字货币,吸引了越来越多用户的关注和投资。因为匿名性和去中心化的特点,比特币也是不法分子常用的洗钱工具。据报道,最近几年比特币已被用于许多案件,包括黑客、暗网市场、资金走私、诈骗和勒索。为了打击此类恶意行为,准确识别比特币地址的类型和比特币交易目的尤为重要。然而,现有的解决方案仅能部分地解决这个问题,并且在识别准确率上表现不佳。在本文中,我们提出了一种基于机器学习的解决方案BATscope,可以准确地识别比特币地址的类型及一些交易的目的(例如,混币交易)。其核心是通过一些可靠的启发式方法和一种新颖的先导预测方法,可以自动化的迭代增加训练集中的比特币地址,从而不断反馈给模型再次训练,稳定提升机器学习模型的性能。评估结果表明,BATscope可以在公开数据集中以0.99的精度识别基于混淆的混币交易,并在识别比特币地址的类型(例如,恶意地址)中达到0.9621/0.9567的Micro/MacroF1分数,远高于现有的解决方案。此外,结果还表明我们的启发式方法可以有效地增强可靠的地址标签数据,先导预测也可以准确的进行纠错并进一步提升模型性能。我们利用BATscope进一步分析了混币交易,揭示了混币行为和恶意地址之间的关系。为了证明其鲁棒性和实用性,我们还使用BATscope来验证已知恶意地址,并帮助执法部门分析未知地址并提供线索。进一步证明在实际应用中,BATscope的结果是可靠的。 |
关键词: *比特币|地址分类|机器学习 |
DOI:10.19363/J.cnki.cn10-1380/tn.2023.07.01 |
Received:December 29, 2021Revised:March 15, 2022 |
基金项目:本课题得到国家重点研发计划资助(No. 2021YFB2701000); 国家自然科学基金资助(No. 61972224, No. U1736209)。 |
|
BATscope: Demystifying Malicious Addresses and Mixing Transactions in Bitcoin |
WONG Taiyu,YIN Tingting,LI Yun,QIN Siliang,REN Xin,LUO Xiapu,WANG Haoyu,YIN Xia,ZHANG Chao |
Department of Computer Science, Tsinghua University, Beijing 100084, China;Institute for Network Science and Cyberspace, Tsinghua University, Beijing 100084, China;School of Cyber Science and Technology. University of Chinese Academy of Sciences, Beijing 100049, China;Department of Software Engineering, Xiamen University, Xiamen 361005, China;Department of Computing, The Hong Kong Polytechnic University, Hong Kong, China;School of Computer Science, Beijing University of Posts and Telecommunications Beijing 100876, China |
Abstract: |
*Bitcoin, the first and the most popular Blockchain-based cryptocurrency, has attracted more and more users and investment. Because of the anonymity and decentralization of the Bitcoin, it has become one of the most common ways for malicious entities to launder money. In recent years, it is reported that Bitcoin has been used as a medium in many illegal actions, including cyberspace hacking, darknet marketplaces, money smuggling, scams, and blackmails. To combat such malicious behaviors, it is crucial to identify the roles of Bitcoin addresses and purposes of Bitcoin transactions of interest. However, existing solutions only partially addressed this problem and had poor performance in recognition. In this paper, we propose a novel machine learning (ML) based solution BATscope to address this problem. BATScope can accurately identify the Bitcoin address type and the purpose of some transaction behaviors (e.g., mixing transactions). At the core, it iteratively and automatically augments the training set of Bitcoin address labels with some reliable heuristics and a novel pilot prediction method, and thereby continuously promotes the ML model's performance. Evaluation results showed that BATscope can recognize obfuscating-based mixing transactions with a precision of 0.99 in the public dataset and recognize the type of Bitcoin addresses (e.g., attackers) with a micro/macro-F1 score of 0.9621/0.9567, much higher than existing solutions. Besides, the result also proved that our reliable heuristics can augment valid address labels with high confidence and pilot prediction corrected mislabeled addresses to further promote model’s performance. We use BATscope to further analyze the mixing transactions in Bitcoin, which revealed the relationship between malicious addresses and mixing transactions. To demonstrate its robustness and usefulness, we also used BATscope to verify known malicious addresses and help law enforcement authorities analyze unknown addresses and close cases. The case studies showed that the result of BATscope is reliable in practical application. |
Key words: *bitcoin|address classification|machine learning |
|
|
|
|
|
|