引用本文: |
-
林子楠,刘杜钢,潘微科,明仲.面向推荐系统中有偏和无偏一元反馈建模的三任务变分自编码器[J].信息安全学报,2021,6(5):110-127 [点击复制]
- LIN Zinan,LIU Dugang,PAN Weike,MING Zhong.Tri-task Variational Autoencoder for Modeling of Biased and Unbiased Unary Feedback in Recommender Systems[J].Journal of Cyber Security,2021,6(5):110-127 [点击复制]
|
|
|
|
本文已被:浏览 5371次 下载 4710次 |
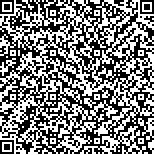 码上扫一扫! |
面向推荐系统中有偏和无偏一元反馈建模的三任务变分自编码器 |
林子楠1,2,3, 刘杜钢1,2,3, 潘微科1,2,3, 明仲1,2,3
|
|
(1.深圳大学大数据系统计算技术国家工程实验室 深圳 中国 518060;2.人工智能与数字经济广东省实验室(深圳) 深圳 中国 518060;3.深圳大学计算机与软件学院 深圳 中国 518060) |
|
摘要: |
一元反馈建模在推荐系统中的应用非常广泛,例如点击预测和购买预测等。然而,推荐系统作为一个闭环的反馈系统,在用户与系统的交互过程中可能存在着多种偏置问题,例如位置偏置、流行偏置等,进而导致用户的反馈数据存在有偏性。现有的大部分推荐模型都只基于这样的有偏数据进行构建,忽略了偏置的影响,进而导致推荐结果是次优的。目前已有的偏置消除方法大致可以分为基于反事实学习的方法、基于启发式的方法和基于无偏数据增强的方法。其中,基于无偏数据增强的方法通常被认为在稳定性和准确性方面表现较好。本文重点研究了推荐系统中一元反馈的偏置问题,通过引入由一种特定策略收集的无偏数据,结合有偏数据进行联合建模,从而得到更准确和无偏的推荐模型。具体而言,本文从多任务学习的角度对问题进行建模,将有偏数据、无偏数据以及它们的并集当作三种相互关联的信号,并设计了三个不同但相关的学习任务。变分自编码器是目前最先进的一元反馈建模方法之一,有着独特的建模方式,从而使得它在很多问题中取得了优越的推荐效果。本文基于变分自编码器提出了一种新的推荐模型,即三任务变分自编码器(Tri-VAE)。该模型包含三个变分自编码器,分别对三种信号进行重构。三个变分自编码器之间共享同一个编码器和同一个解码器。此外,本文还设计了特征校正模块和标签增强模块以加强任务之间的关联。其中,特征校正模块用来校正用户的潜在特征,得到更无偏的潜在特征,进而从潜在特征的角度缓解偏置的影响。标签增强模块用于生成可靠性较高的伪标签并加以利用,进而更有效地利用无偏数据中的信息。在Yahoo!R3和Coat Shopping两个公开数据集上的实验结果表明,所提出的模型相比于目前最新的基线模型在绝大多数情况下取得了显著的效果提升。为了进一步研究所提出的模型,本文进行了消融实验、超参数敏感性分析和收敛性分析,还对特征校正模块的有效性进行了探讨。 |
关键词: 偏置问题 联合建模 多任务学习 变分自编码器 推荐系统 |
DOI:10.19363/J.cnki.cn10-1380/tn.2021.09.09 |
投稿时间:2021-04-26修订日期:2021-07-30 |
基金项目:本课题得到国家自然科学基金重点项目(No.61836005)和国家自然科学基金面上项目(No.61872249)资助。 |
|
Tri-task Variational Autoencoder for Modeling of Biased and Unbiased Unary Feedback in Recommender Systems |
LIN Zinan1,2,3, LIU Dugang1,2,3, PAN Weike1,2,3, MING Zhong1,2,3
|
(1.National Engineering Laboratory for Big Data System Computing Technology, Shenzhen University, Shenzhen 518060, China;2.Guangdong Laboratory for Artificial Intelligence and Digital Economy(SZ), Shenzhen 518060, China;3.College of Computer Science and Software Engineering, Shenzhen University, Shenzhen 518060, China) |
Abstract: |
Modeling of unary feedback is widely used in recommender systems for click prediction and purchase prediction. However, as a closed-loop feedback system, a recommender system may have various bias issues during the user-system interaction, such as position bias and popularity bias, leading to biased user feedback data. Most of the existing recommendation models are constructed based on such biased data, ignoring the influence of the bias, which leads to sub-optimal recommendation results. The existing works for bias elimination can be roughly divided into three categories, i.e., counterfactual learning-based methods, heuristic-based methods and unbiased data augmentation methods. Among them, the unbiased data augmentation methods are usually considered to be of better stability and accuracy. In this paper, we focus on the bias problem of unary feedback in recommender systems. By introducing an unbiased data collected by a specific policy and then jointly modeling it with the biased data, we are able to learn a more accurate and unbiased recommendation model. Specifically, from the perspective of multi-task learning, we regard the biased data, the unbiased data and their union as three correlated signals, and then design three different but related preference learning tasks. Variational autoencoder is a state-of-the-art method for modeling unary feedback. Because of its unique modeling ideas, it has achieved superior recommendation performance in many cases. Based on variational autoencoder, we design a novel recommendation model called tri-task variational autoencoder (Tri-VAE). Our proposed model contains three variational autoencoders that reconstruct three different signals, respectively. All variational autoencoders share the same encoder and the same decoder. In addition, we also design a feature calibration module and a label enhancement module to strengthen the association between the three tasks. In particular, the feature calibration module is used to calibrate the latent features of users to obtain more unbiased latent features, which in turn mitigates the impact of bias from the perspective of latent features. The label enhancement module is implemented to generate pseudo labels with high reliability, which can be exploited to make our proposed model more effective in exploiting the information in unbiased data. The experimental results on two public datasets, including Yahoo! R3 and Coat Shopping, show that the proposed model achieves significantly better performance compared with the latest baseline models in most cases. In order to further study our proposed model, we conduct ablation studies, hyper-parameter sensitivity analysis and convergence analysis and discuss the effectiveness of the feature calibration module. |
Key words: bias issue joint modeling multi-task learning variational autoencoder recommender system |
|
|
|
|
|