引用本文: |
-
王豪,许强,张清华,李开菊.LFDP:融合低频信息的差分隐私鲁棒性增强方法[J].信息安全学报,2025,10(1):47-60 [点击复制]
- WANG Hao,XU Qiang,ZHANG Qinghua,LI Kaiju.LFDP: A Differentially Private Robustness Augmentation Method Combining Low-Frequency Information[J].Journal of Cyber Security,2025,10(1):47-60 [点击复制]
|
|
|
|
本文已被:浏览 100次 下载 36次 |
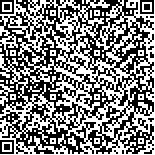 码上扫一扫! |
LFDP:融合低频信息的差分隐私鲁棒性增强方法 |
王豪1,2, 许强3, 张清华4, 李开菊1,5
|
|
(1.重庆邮电大学 计算机科学与技术学院 重庆 中国 400065;2.旅游多源数据感知与决策技术文化和旅游部重点实验室 重庆 中国 400065;3.香港城市大学 电机工程系 香港 中国 999077;4.重庆邮电大学 计算智能重庆市重点实验室 重庆 中国 400065;5.重庆大学 计算机学院 重庆 中国 400044) |
|
摘要: |
机器学习模型由于其预测和分类的高精度和各种应用场景的普适性,在图像处理、自动驾驶、自然语言处理等领域得到广泛应用。但机器学习模型容易遭受对抗样本攻击,在遭受对抗样本攻击时,预测和分类的精度会大幅下降。目前,数据增强方法通过改变或者扰动原始图像的方式,使得机器学习模型具有更强的泛化能力,在保护隐私的同时,能够增强其抵御对抗样本攻击的鲁棒性,是当前机器学习模型鲁棒性增强的主流方法之一。但基于差分隐私的鲁棒性增强方法面临加入的高频噪声容易被滤除,导致鲁棒性增强效果下降的问题。针对这一问题,结合信号处理的知识,本文从频域角度阐述差分隐私能够增强机器学习模型鲁棒性的原理,从理论上证明其有效性。设计了一种高频噪声滤波器HFNF,能够将差分隐私加入的高频高斯噪声滤除,使得差分隐私的鲁棒性增强效果下降,从理论上分析差分隐私鲁棒性增强方法存在缺陷的原因。提出了一种普适的融合低频信息的差分隐私鲁棒性增强算法LFDP,通过对图像不同频域部分加入生成的高低频噪声,即使存在高频噪声滤波攻击,仍然能够保证模型的鲁棒性,弥补了差分隐私原有高频高斯噪声的不足。从理论上分析并给出所提出方案的鲁棒性和误差边界,并在实际的数据集中进行测试。实验结果表明,与直接加入高频噪声的差分隐私鲁棒性增强方法相比,LFDP在不增大噪声尺度的同时能够起到更好的鲁棒性增强效果。 |
关键词: 机器学习 鲁棒性 差分隐私 低频噪声 |
DOI:10.19363/J.cnki.cn10-1380/tn.2025.01.04 |
投稿时间:2022-12-11修订日期:2023-03-11 |
基金项目:本课题得到国家自然科学基金(No.42001398,No.62402150,No.62276038)、国家重点研发计划课题(No.2020YFC2003502)、重庆市教委科学技术研究重点项目(No.KJZD-K202300601)、贵州财经大学引进人才科研启动基金(No.2023YJ10)、旅游多源数据感知与决策技术文化和旅游部重点实验室开放基金资助项目(No.TMDPD-2023N-002)、贵州省教育厅青年科技成长项目(黔教技[2024]86)、重庆邮电大学计算机学院人才梯队提升计划项目(No.JKY-202423)、贵州省科技计划项目(黔科合成果[2024]重大018)资助 |
|
LFDP: A Differentially Private Robustness Augmentation Method Combining Low-Frequency Information |
WANG Hao1,2, XU Qiang3, ZHANG Qinghua4, LI Kaiju1,5
|
(1.College of Computer Science and Technology, Chongqing University of Posts and Telecommunications, Chongqing 400065, China;2.Key Laboratory of Tourism Multisource Data Perception and Decision, Ministry of Culture and Tourism, Chongqing University of Posts and Telecommunications, Chongqing 400065, China;3.Department of Electrical Engineering, City University of Hong Kong, Hongkong 999077, China;4.Key Laboratory of Big Data Intelligent Computing, Chongqing University of Posts and Telecommunications, Chongqing 400065, China;5.College of Computer Science, Chongqing University, Chongqing 400044, China) |
Abstract: |
Machine learning model has been widely used in image processing, automatic driving, natural language processing and other fields because of its high accuracy of prediction and classification and the universality of various application scenarios. However, the machine learning model is vulnerable to counter sample attacks. When it is attacked by counter sample attacks, the accuracy of prediction and classification will be greatly reduced. At present, the data enhancement method makes the machine learning model have stronger generalization ability by changing or disturbing the original image, and can enhance its robustness against sample attacks while protecting privacy, which is one of the mainstream methods for enhancing the robustness of machine learning models. However, the robustness enhancement method based on differential privacy is faced with the problem that the added high-frequency noise is easy to be filtered out, resulting in a decline in the robustness enhancement effect. Aiming at this problem, combined with the knowledge of signal processing, this paper expounds the principle that differential privacy can enhance the robustness of machine learning models from the perspective of frequency domain, and proves its effectiveness in theory. A high frequency noise filter HFNF is designed, which can filter out the high frequency Gaussian noise added by differential privacy and reduce the robustness enhancement effect of differential privacy. The reason for the defects of the robustness enhancement method of differential privacy is analyzed theoretically. This paper proposes a universal differential privacy robustness enhancement algorithm LFDP, which fuses low frequency information. By adding high and low frequency noise generated in different frequency domain parts of the image, even if there is high frequency noise filtering attack, the robustness of the model can still be guaranteed, making up for the deficiency of the original high frequency Gaussian noise in differential privacy. The robustness and error boundary of the proposed scheme are theoretically analyzed and given, and tested in actual data sets. The experimental results show that compared with the difference privacy robustness enhancement method directly adding high-frequency noise, LFDP can play a better robustness enhancement effect without increasing the noise scale. |
Key words: machine learning robustness differential privacy low-frequency noise |
|
|
|
|
|