引用本文: |
-
韩蒙,俞伟平,周依云,杜文涛,孙彦斌,林昶廷.基于特征分布差异的对抗样本检测[J].信息安全学报,2023,8(3):1-11 [点击复制]
- HAN Meng,YU Weiping,ZHOU Yiyun,DU Wentao,SUN Yanbin,LIN Changting.Exploiting Feature Space Divergence For Adversarial Example Detection[J].Journal of Cyber Security,2023,8(3):1-11 [点击复制]
|
|
|
|
本文已被:浏览 10159次 下载 6887次 |
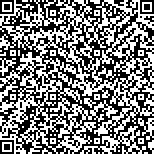 码上扫一扫! |
基于特征分布差异的对抗样本检测 |
韩蒙1,2, 俞伟平3, 周依云4, 杜文涛1, 孙彦斌5, 林昶廷1,2
|
|
(1.浙江大学 杭州 中国 310007;2.浙江君同智能科技有限责任公司 杭州中国 310051;3.杭州涂鸦科技有限公司 杭州 中国 310010;4.CareerBuilder 芝加哥 美国 60601;5.广州大学 广州 中国 510006) |
|
摘要: |
诸多神经网络模型已被证明极易遭受对抗样本攻击。对抗样本则是攻击者为模型所恶意构建的输入,通过对原始样本输入添加轻微的扰动,导致其极易被机器学习模型错误分类。这些对抗样本会对日常生活中的高要求和关键应用的安全构成严重威胁,如自动驾驶、监控系统和生物识别验证等应用。研究表明在模型的训练期间,检测对抗样本方式相比通过增强模型来预防对抗样本攻击更为有效,且训练期间神经网络模型的中间隐层可以捕获并抽象样本信息,使对抗样本与干净样本更容易被模型所区分。因此,本文针对神经网络模型中的不同隐藏层,其对抗样本输入和原始自然输入的隐层表示进行统计特征差异进行研究。本文研究表明,统计差异可以在不同层之间进行区别。本文通过确定最有效层识别对抗样本和原始自然训练数据集统计特征之间的差异,并采用异常值检测方法,设计一种基于特征分布的对抗样本检测框架。该框架可以分为广义对抗样本检测方法和条件对抗样本检测方法,前者通过在每个隐层中提取学习到的训练数据表示,得到统计特征后,计算测试集的异常值分数,后者则通过深层神经网络模型对测试数据的预测结果比较,得到对应训练数据的统计特征。本文所计算的统计特征包括到原点的范数距离L2和样本协方差矩阵的顶奇异向量的相关性。实验结果显示了两种检测方法均可以利用隐层信息检测出对抗样本,且对由不同攻击产生的对抗样本均具有较好的检测效果,证明了本文所提的检测框架在检测对抗样本中的有效性。 |
关键词: 神经网络|特征分布差异|对抗样本检测|异常值检测 |
DOI:10.19363/J.cnki.cn10-1380/tn.2023.05.01 |
投稿时间:2022-08-09修订日期:2022-12-15 |
基金项目:本文研究成果得到国家自然科学基金项目(No. 62072404), CCF-蚂蚁科研基金(CCF-AFSG No. RF20220003),杭州市领军型创新创业团队(No. TD2022011)资助。 |
|
Exploiting Feature Space Divergence For Adversarial Example Detection |
HAN Meng1,2, YU Weiping3, ZHOU Yiyun4, DU Wentao1, SUN Yanbin5, LIN Changting1,2
|
(1.Zhejiang University, Hangzhou 310007, China;2.Gentel. ai, Hangzhou 310051, China;3.Tuya Inc., Hangzhou 310010, China;4.CareerBuilder, IL, 60601, USA;5.Guangzhou University, Guangzhou 510006, China) |
Abstract: |
Neural network models have been shown to be vulnerable to adversarial examples, which are the maliciously crafted inputs with adding slight perturbation to the original natural inputs, resulting in incorrect classification by the ML model. Such adversarial samples threaten the security of high requirements and key applications in daily life, such as autonomous driving, surveillance systems, and biometric authentication. Recent works have shown detecting adversarial examples can be more effective than preventing them by enhancing models during training time. Moreover, the neural network model is more easier to distinguish adversarial samples from original natural samples as its middle hidden layer capture and extract sample information during training time. Therefore, we investigate the statistical divergence of hidden representations between adversarial inputs and benign inputs on different layers in neural networks in this study. Our results show that this divergence can vary among different layers. By identifying the most effective layers for identifying the divergence and the statistical representation distribution of the benign training datasets, a framework for adversarial samples detection using feature distribution is proposed in this paper. The framework can be divided into generalized adversarial samples detection method and conditional adversarial samples detection method. The former calculates the outlier score of the test set after obtaining statistical features by extracting the learned training data representation from each hidden layer. The latter obtains the statistical features of the corresponding training data by comparing the prediction results of deep neural network models. The calculated statistical features include the L2 norm distance from the origin and the correlation with the top eigenvalue of the sample covariance matrix. Our experiment results show that both detection methods can detect adversarial samples using hidden layer information and have good detection effects on adversarial samples generated by different attacks, thereby demonstrating the effectiveness of the proposed detection framework in detecting adversarial samples. |
Key words: neural network|feature space divergence|adversarial example detection|outlier detection |
|
|
|
|
|