引用本文: |
-
胡智超,余翔湛,刘立坤,张宇,于海宁.基于关系挖掘和注意力的多维时序异常检测[J].信息安全学报,2024,9(6):100-113 [点击复制]
- HU Zhichao,YU Xiangzhan,LIU Likun,ZHANG Yu,YU Haining.Relation Mining and Attention Based Anomaly Detection for Multivariate Time Series[J].Journal of Cyber Security,2024,9(6):100-113 [点击复制]
|
|
|
|
本文已被:浏览 698次 下载 299次 |
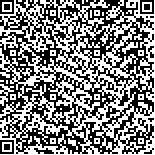 码上扫一扫! |
基于关系挖掘和注意力的多维时序异常检测 |
胡智超, 余翔湛, 刘立坤, 张宇, 于海宁
|
|
(网络空间安全学院 哈尔滨工业大学 哈尔滨 中国 150001) |
|
摘要: |
在当前信息化的时代,多维时序数据的异常检测应用广泛,常用于云服务器、在线服务、系统日志、工业物联网以及智能交通等场景下的状态监控和数据分析中。相比于单一维度的时间序列,多维时序更加符合实际的场景需求。比如云服务器的关键性能指标,包括主机CPU、内存、磁盘IO以及网络流量等,均从不同角度反应了系统状态,同时彼此之间又存在着关系。传统的时序异常检测方法对这种影响关系考虑不足或者难以高效挖掘这种序列间的隐式关系,给传统方法在多维时序数据中的应用带来了挑战。本文针对现有方法存在的不足,提出了基于关系挖掘和注意力机制的异常检测算法TSAN。该方法首先提出了端到端的序列关系挖掘方法,通过节点嵌入表示的相似性和图结构来挖掘序列之间关系,并结合top-k和阈值机制来修剪关系图确保其简洁性,接着利用因果推断生成序列间的因果关系图作为遮罩层,提高关系图的可解释性和有效性。然后,TSAN设计了时空注意力网络,使用时间和空间维度的联合注意力机制来处理混合时空上下文,用于关系挖掘后的多维时序预测。最后,提出了异常阈值自动计算方法,减少了多维时间序列场景下的超参设置,并且引入最大异常容忍率来排除异常数据的影响,提高了算法的鲁棒性。从实验结果可以看出,TSAN在数据集MSL和SMD上取得了最优的F1值,相比次优方法分别提升了0.9%(MSL)和2.3%(SMD),并且在所有对比方法中具有最小的跨数据集性能波动,说明了TSAN对多维时序数据的异常检测是有效且稳定的。 |
关键词: 异常检测 多维时间序列 关系挖掘 注意力 机器学习 |
DOI:10.19363/J.cnki.cn10-1380/tn.2024.11.07 |
投稿时间:2022-10-28修订日期:2022-12-13 |
基金项目:本课题得到国家重点研发计划项目(No.2018YFB1800702)资助。 |
|
Relation Mining and Attention Based Anomaly Detection for Multivariate Time Series |
HU Zhichao, YU Xiangzhan, LIU Likun, ZHANG Yu, YU Haining
|
(School of Cyberspace Science, Harbin Institute of Technology, Harbin 150001, China) |
Abstract: |
In the current era of information technology, anomaly detection of multivariate time series is widely used for status monitoring and data analysis in scenarios such as cloud servers, online services, system logs, industrial IoT and intelligent transportation. Compared to single dimensional time series, multivariate time series are more in line with the actual scenario requirements. For example, the key performance indicators of cloud servers, including CPU, memory, disk IO and network traffic information, all reflect the system status from different perspectives and have a relationship with each other. However, traditional anomaly detection methods do not sufficiently consider such influential relationships or are difficult to efficiently mine the implicit relationships between sequences, posing a challenge for the application of traditional methods in multivariate time series. To addresses these limitations, this paper proposes TSAN, an anomaly detection method based on relation mining and attention mechanism. The method first introduces an end-to-end sequence relation mining method. It mines the relation between sequences through the similarity of node embedding and graph structure, and combines top-k and threshold mechanisms to prune the relationship graph to ensure its simplicity, followed by using causal inference to generate the causal graph of sequences as mask layer to improve the interpretability and effectiveness. TSAN then designs a temporal-spatial attention network using a joint attention mechanism to handle the mixed contexts for multivariate timeseries prediction after relation mining. Finally, an automatic calculation method for anomaly threshold is designed to reduce the hyper-parameter settings in multivariate time series scenarios. Besides, TSAN introduces the maximum anomaly tolerance rate to reduce the influence of anomalous data and improves the robustness. From the experimental results, it can be seen that TSAN achieves the best F1 score on the MSL and SMD datasets, with an improvement of 0.9% (MSL) and 2.3% (SMD) respectively compared to the sub-optimal methods and has the smallest cross-dataset performance fluctuations among all the compared methods, indicating that TSAN is effective and stable for anomaly detection of multivariate time series. |
Key words: anomaly detection multivariate time series relation mining attention machine learning |
|
|
|
|
|