引用本文: |
-
王旭仁,魏欣欣,王媛媛,姜政伟,江钧,杨沛安,刘润时.网络威胁情报实体识别研究综述[J].信息安全学报,2024,9(6):74-99 [点击复制]
- WANG Xuren,WEI Xinxin,WANG Yuanyuan,JIANG Zhengwei,JIANG Jun,YANG Peian,LIU Runshi.A Survey of Cyber Threat Intelligence Entity Recognition Research[J].Journal of Cyber Security,2024,9(6):74-99 [点击复制]
|
|
|
|
本文已被:浏览 3742次 下载 1835次 |
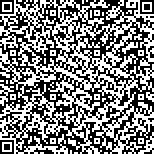 码上扫一扫! |
网络威胁情报实体识别研究综述 |
王旭仁1,2, 魏欣欣1,2, 王媛媛3, 姜政伟2,4, 江钧2,4, 杨沛安2,4, 刘润时1
|
|
(1.首都师范大学信息工程学院 北京 中国 100048;2.中国科学院信息工程研究所 中国科学院网络测评技术重点实验室 北京 中国 100093;31005 部队 北京 中国 100089;4.中国科学院大学网络空间安全学院 北京 中国 100049) |
|
摘要: |
由于网络环境愈发复杂,网络安全形势日渐严峻,保护网络免受外来攻击成为一项重要的工作。为了使网络空间攻防技术变为主动防御的形式,网络威胁情报应运而生。通过对网络威胁情报进行分析和检测,搜集情报证据,能够预防攻击行为的发生。因此,通过共享网络威胁情报来抵御网络攻击变得愈发重要。然而,网络威胁情报通常以非结构化的形式共享,将其转化为半结构化或结构化数据对后续很多任务来讲尤为重要,命名实体识别技术能够实现这一点。虽然在通用领域的命名实体识别已经取得了非常不错的成果,但在网络威胁情报领域却仍然存在很多问题。本文首先介绍威胁情报相关背景,及其与命名实体识别之间的联系。然后根据命名实体识别技术发展的时间顺序总结基于规则和词典的实体识别技术、基于无监督学习的实体识别技术、基于特征的监督学习实体识别技术、基于深度学习的实体识别技术等,全面总结威胁情报领域命名实体识别的研究现状和未来的发展方向。最后,对比研究威胁情报领域命名实体识别所使用的语料库,使用SOTA深度学习方法进行实验,分析总结出威胁情报领域数据集所存在的问题。提出的BBC (BERT-BiGRU-CRF)深度学习实体识别模型具有最好的实验效果,在AutoLabel数据集、DNRTI数据集、CTIReports数据集,以及APTNER数据集上分别达到97.36%、90.40%、82.87%、73.91%的F1值。 |
关键词: 命名实体识别 网络威胁情报 深度学习 网络威胁情报数据集 |
DOI:10.19363/J.cnki.cn10-1380/tn.2024.11.06 |
投稿时间:2023-02-04修订日期:2023-05-15 |
基金项目:本课题得到中国科学院青年创新促进会(No.2020166);中科院战略先导项目(No.XDC02030200)的资助。 |
|
A Survey of Cyber Threat Intelligence Entity Recognition Research |
WANG Xuren1,2, WEI Xinxin1,2, WANG Yuanyuan3, JIANG Zhengwei2,4, JIANG Jun2,4, YANG Peian2,4, LIU Runshi1
|
(1.Information Engineering College, Capital Normal University, Beijing 100048, China;2.Key Laboratory of Network Assessment Technology, Institute of Information Engineering, Chinese Academy of Sciences, Beijing 100093, China;3.Unit 31005 of PLA, Beijing 100089, China;4.School of Cyber Security, University of Chinese Academy of Sciences, Beijing 100049, China) |
Abstract: |
As the network environment becomes increasingly complex, the security landscape is growing more severe, making the protection of networks from external attacks a crucial task. In order to transform cybersecurity from a reactive defense approach to proactive defense, Cyber Threat Intelligence (CTI) has emerged. By analyzing and detecting CTI, gathering intelligence evidence, potential attacks can be prevented. Therefore, sharing CTI to defend against cyber-attacks has become increasingly important. However, CTI is often shared in an unstructured format, making its conversion to semi-structured or structured data essential for many subsequent tasks. Named Entity Recognition (NER) technology can facilitate this transformation. Although NER has achieved considerable success in general domains, many challenges remain in the field of CTI. This article first introduces the background of threat intelligence and its connection to NER. Then, it summarizes NER technologies in chronological order, covering rule-based and dictionary-based NER, unsupervised learning methods, feature-based supervised learning methods, and deep learning-based NER. It provides a comprehensive overview of the current research status and future directions of NER in the CTI field. Lastly, a comparative study of the corpora used for NER in CTI is conducted, followed by experiments using state-of-the-art (SOTA) deep learning methods. The analysis identifies issues present in CTI datasets. The proposed BBC (BERT-BiGRU-CRF) deep learning entity recognition model achieves the best experimental results, with F1 scores of 97.36%, 90.40%, 82.87%, and 73.91% on the AutoLabel, DNRTI, CTIReports, and APTNER datasets, respectively. |
Key words: named entity recognition Cyber Threat Intelligence (CTI) deep learning CTI datasets |
|
|
|
|
|