引用本文: |
-
韩瑶鹏,王璐,姜波,卢志刚,姜政伟,刘玉岭.基于预训练模型的网络空间安全命名实体识别方法[J].信息安全学报,2025,10(1):194-204 [点击复制]
- HAN Yaopeng,WANG Lu,JIANG Bo,LU Zhigang,JIANG Zhengwei,LIU Yuling.Cybersecurity Named Entity Recognition using the Pre-trained Model[J].Journal of Cyber Security,2025,10(1):194-204 [点击复制]
|
|
|
|
本文已被:浏览 756次 下载 223次 |
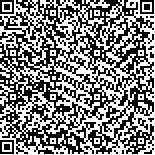 码上扫一扫! |
基于预训练模型的网络空间安全命名实体识别方法 |
韩瑶鹏1,2, 王璐1,2, 姜波1,2, 卢志刚1,2, 姜政伟1,2, 刘玉岭1,2
|
|
(1.中国科学院信息工程研究所 北京 中国 100093;2.中国科学院大学 网络空间安全学院 北京 中国 100049) |
|
摘要: |
随着网络空间安全文档数量的快速增长,网络空间安全领域命名实体识别变的越来越重要。与通用领域命名实体识别任务相比,网络空间安全领域的命名实体识别面临许多挑战。例如网络空间安全实体类型多样、新词语经常作为新的实体出现并引起超出词表(out-of-vocabulary,OOV)的问题。现有的深度学习识别模型(如循环神经网络、卷积神经网络)的性能不足以应对这些挑战。随着预训练模型的快速发展,它已被广泛用于许多任务中并获得了最优的表现。但是,在网络空间安全命名实体识别领域,很少有关于预训练模型的研究。本文提出了两个基于预训练pre-training of deep bidirectional transformers (BERT)模型的网络空间安全命名实体识别模型来从网络空间安全文本中提取安全实体,分别称为“First Subword Replaced (FSR)”和“MaskedCross-Entropy Loss (MCEL)”。FSR模型和MCEL模型还可以解决因BERT使用WordPiece分词器引起的子词和标签之间的不匹配问题。本文基于真实的网络空间安全文本语料库进行了充分的实验。结果表明,本文提出基于预训练的模型在网络空间安全数据集上的F1值比之前的最优模型高了1.88%。 |
关键词: 网络空间安全 命名实体识别 预训练模型 |
DOI:10.19363/J.cnki.cn10-1380/tn.2025.01.14 |
投稿时间:2020-09-23修订日期:2021-03-26 |
基金项目:本论文得到国家重点研发计划(No.2021YFF0307203,No.2019QY1303,No.2019QY1302)、中国科学院战略性先导C类(No.XDC02040100),基础加强计划技术领域基金(No.2021-JCJQ-JJ-0908)、国家自然科学青年基金(No.61902376)和信息安全等级保护关键技术国家工程实验室(公安部第三研究所)开放课题(No.C21640-3)的资助。这项工作也得到了中国科学院网络评估技术重点实验室和北京市网络安全与保护技术重点实验室的部分支持。 |
|
Cybersecurity Named Entity Recognition using the Pre-trained Model |
HAN Yaopeng1,2, WANG Lu1,2, JIANG Bo1,2, LU Zhigang1,2, JIANG Zhengwei1,2, LIU Yuling1,2
|
(1.Institute of Information Engineering, Chinese Academy of Sciences, Beijing 100093, China;2.School of Cyber Security, University of Chinese Academy of Sciences, Beijing 100049, China) |
Abstract: |
Cybersecurity named entity recognition (NER) is becoming increasingly important as the number of cybersecurity documents rapidly grows. Compared with general domain NER tasks, cybersecurity-domain NER faces many challenges. There are many types of security entities, and new words often appear as entities causing out-of-vocabulary (OOV) problems. Existing deep learning recognition models (RNNs, CNNs) do not perform enough to deal with these challenges. With the rapid development of the pre-trained model, it is widely used in many tasks and achieved state-of-the-art performance. However, in the domain of cybersecurity NER, there are few studies on the pre-trained model. This paper proposes two cybersecurity NER models, named First Subword Replaced (FSR) and Masked Cross-Entropy Loss (MCEL), based on the pre-trained BERT (pre-training of deep bidirectional transformers) model to extract security entities from the cybersecurity dataset. The FSR and MCEL models can also deal with the mismatch between the subwords and labels caused by BERT using WordPiece tokenizer. This paper conducts extensive experiments on a real-world cybersecurity text corpora. The results show that the pre-trained model proposed in this paper outperforms the previous state-of-the-art method by 1.88% F1 score on the cybersecurity dataset. |
Key words: cybersecurity named entity recognition the pre-trained model |
|
|
|
|
|