引用本文: |
-
石瑞生,付彤,林子丁,兰丽娜,姜宁.基于联邦学习的动态信任评估身份认证方法[J].信息安全学报,2025,10(2):30-47 [点击复制]
- SHI Ruisheng,FU Tong,LIN Ziding,LAN Lina,JIANG Ning.Research on Dynamic Trust Evaluation Method Based on Federated Learning[J].Journal of Cyber Security,2025,10(2):30-47 [点击复制]
|
|
|
|
本文已被:浏览 1196次 下载 316次 |
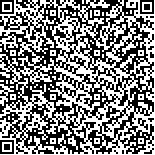 码上扫一扫! |
基于联邦学习的动态信任评估身份认证方法 |
石瑞生1,2, 付彤1, 林子丁1, 兰丽娜3, 姜宁1
|
|
(1.北京邮电大学 网络空间安全学院 北京 中国 100876;2.北京邮电大学 可信分布式计算与服务教育部重点实验室 北京 中国 100876;3.北京邮电大学 人文学院 北京 中国 100876) |
|
摘要: |
随着云计算、BYOD (Bring your own device)的流行,企业信息系统呈现出开放与动态互联的特征,这种趋势使得基于动态信任评估的零信任安全架构开始取代基于边界信任的一次性身份认证模式,成为工业界与学术界关注的研究热点。动态信任评估模型为零信任架构提供持续信任评估的能力,可以对企业信息系统的安全性和隐私性进行有效的保护。然而,训练动态信任评估模型面临两个现实挑战:1)很多企业的用户异常登录行为数据很少,影响模型的训练效果,导致信任评估模型准确性不高,不利于身份认证系统的可靠性;2)用户行为数据中包含着用户的隐私信息,泄漏用户隐私的法律风险使得企业不愿意共享用户异常登录行为数据。针对这些问题,本文提出了一种基于联邦学习的动态信任评估身份认证方法,使得各个平台在不泄漏原始用户数据的情况下达到联合训练模型的目的,进而提高各平台身份认证系统的安全性。在假设各个平台提供了用户的行为原始数据的前提下,本方案会根据不同特征的实际含义提取离散型用户行为数据的统计学特征,并选取与风险用户相关性高的特征。为了保证数据安全性和训练数据的规模,本方法采用联邦学习技术联合多个企业进行训练,从而得到动态信任评估层的核心模型,其误识率和拒识率相较于单一平台有了一定的提升。通过该方案,身份认证系统可以在不泄露用户敏感信息的情况下,对用户身份进行有效评估,进而提升身份认证系统安全性和用户体验。本文还对不同的支持横向联邦学习的机器学习算法应用于动态信任评估模型的效果进行了比较,实验结果表明了在基于联邦学习的动态身份认证模型中使用SVM作为机器学习训练方法的效果优于其他机器学习训练方法。最后,本文从安全性和隐私性的角度出发还对动态信任评估系统自身以及联邦学习带来的安全性和隐私性的影响做了讨论。 |
关键词: 联邦学习 动态信任评估 网络安全 身份认证 |
DOI:10.19363/J.cnki.cn10-1380/tn.2025.03.03 |
投稿时间:2023-04-02修订日期:2023-07-25 |
基金项目:本课题得到北京市自然科学基金(No. M21037)、国家重点研发计划项目(No. 2022YFF0902701)、工业与信息化部“2022 年工业互联网公共服务平台-面向工业互联网的虚拟货币挖矿治理公共服务平台项目”、教育部“虚拟货币挖矿行为监管平台研发与应用重大攻关项目”、中国-中东欧国家高校联合教育项目(No. 2022172)、高等学校学科创新引智计划(No. B21049)、北京邮电大学研究生创新创业项目(No. 2025-YC-T020)资助。 |
|
Research on Dynamic Trust Evaluation Method Based on Federated Learning |
SHI Ruisheng1,2, FU Tong1, LIN Ziding1, LAN Lina3, JIANG Ning1
|
(1.School of Cyberspace Security, Beijing University of Posts and Telecommunications, Beijing 100876, China;2.Key Laboratory of Trustworthy Distributed Computing and Service, Ministry of Education, Beijing University of Posts and Telecommunications, Beijing 100876, China;3.School of Humanities, Beijing University of Posts and Telecommunications, Beijing 100876, China) |
Abstract: |
With the popularity of cloud computing and Bring Your Own Device (BYOD), enterprise information systems exhibit open and dynamic interconnection features. This trend has led to the replacement of the one-time identity authentication mode based on boundary trust with the zero-trust security architecture based on dynamic trust evaluation, which has become a research hotspot in industry and academia. The dynamic trust evaluation model provides continuous trust evaluation for the zero-trust architecture, which can effectively protect the security and privacy of enterprise information systems. However, training dynamic trust evaluation models faces two practical challenges: 1) many enterprises have limited abnormal login behavior data, which affects the training effectiveness of the model and leads to low accuracy of the trust evaluation model, which is not conducive to the reliability of the identity authentication system; and 2) user behavior data contains users’ privacy information, and the legal risk of privacy leakage makes enterprises unwilling to share abnormal login behavior data. To address these issues, this paper proposes a dynamic trust evaluation method based on federated learning, which enables various platforms to achieve joint training of the model without leaking the original user data, thereby improving the security of identity authentication systems on various platforms. Assuming that various platforms provide users’ raw behavioral data, this approach extracts statistical features of discrete user behavior data based on the actual meaning of different features and selects features with high relevance to risky users. To ensure data security and training data scale, this method uses federated learning technology to train multiple enterprises together to obtain the core model of the dynamic trust evaluation layer, achieving 0.205 system false acceptance rate and 0.192 system false rejection rate, with improved accuracy compared to a single platform. Through this approach, the identity authentication system can effectively evaluate user identity without leaking sensitive information, thereby improving system security and user experience. This paper also compares the effects of different machine learning algorithms supporting horizontal federated learning applied to dynamic trust evaluation models. The experimental results show that using SVM as the machine learning training method in the dynamic identity authentication model based on federated learning is more effective than other methods. Finally, this paper discusses the security and privacy impact of the dynamic trust evaluation system itself and the federated learning from the perspective of security and privacy. |
Key words: federated learning dynamic trust assessment network security identity authentication |
|
|
|
|
|